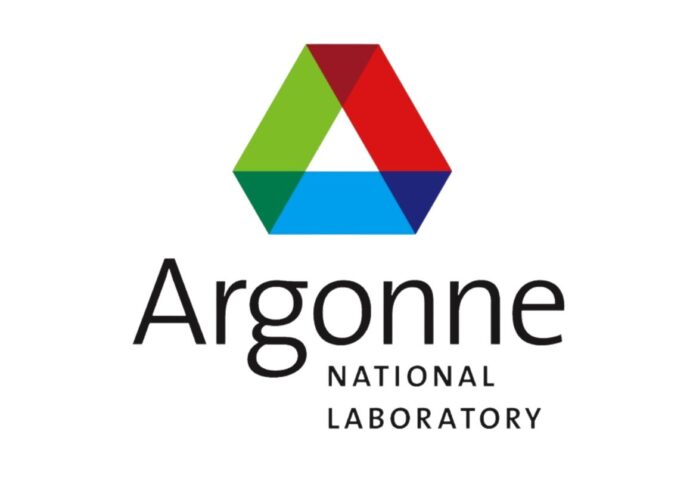
A research team led by the US Department of Energy’s (DOE) Argonne National Laboratory and the University of Virginia (UVA) has developed various imaging and machine-learning techniques to detect and predict the formation of pores in 3D-printed metals in real time with near-perfect accuracy.
Published in the journal Science, the study titled “Machine learning–aided real-time detection of keyhole pore generation in laser powder bed fusion” could potentially transform and advance the additive manufacturing process, according to the researchers.
The metal samples used in the research were created using a process called laser powder bed fusion, in which metal powder is heated by a laser and then melted into the proper shape.
The researchers said this method often results in the development of pores that can impair a part’s performance.
The research team explained that many additive manufacturing machines feature thermal imaging sensors that keep track of the build process, however since these sensors only photograph the surface of the items being built, they may overlook the development of pores.
Thus, employing powerful X-ray beams is the only technique to directly identify pores inside solid, metal objects, the research team noted.
“Our X-ray beams are so intense that we can image more than a million frames per second,” said Samuel Clark, an assistant physicist at Argonne.
The researchers then were able to observe pore development in real-time thanks to these photos, and the scientists found that pores produced within a sample produce separate thermal fingerprints at the surface that thermal cameras can identify by comparing X-ray and thermal images.
Furthermore, the research team used solely thermal pictures to train a machine-learning model to forecast the development of pores within 3D metals.
They used information from the X-ray scans, which they knew accurately depicted the formation of pores, to validate the model.
The scientists then put the model to the test by predicting pore development and detecting thermal signals in unlabeled samples.
“The [Advanced Photon Source] offered the 100% accurate ground truth that allowed us to achieve perfect prediction of pore generation with our model,” said Tao Sun, an associate professor at UVA.
Meanwhile, Argonne physicist Kamel Fezzaa remarked that their approach may easily be adopted in commercial systems.
“With only a thermal camera, the machines should be able to detect when and where pores are generated during the printing process and adjust their parameters accordingly,” Fezzaa noted.
The researchers will now look into sensors that can detect other types of defects that occur during the additive manufacturing process.
“In the end, we want to develop a comprehensive system that can tell you not only where you possibly have defects, but also what exactly the defect is and how it might be fixed,” Sun concluded.