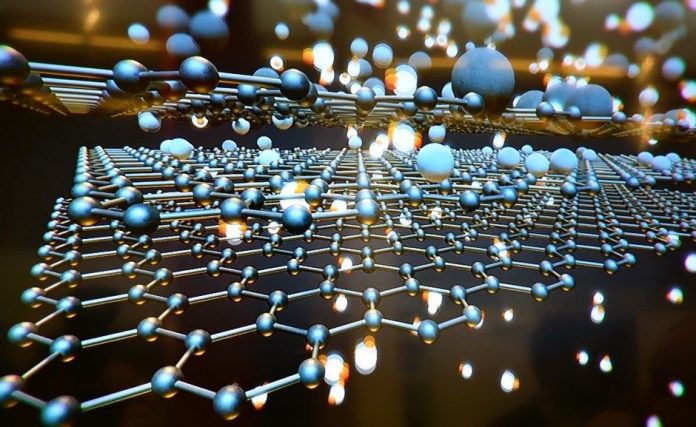
A team of Monash University engineers led by Professor Mainak Majumder have developed a novel technology capable of identifying high quality graphene cheaper, faster and more accurately than current methods.
Using the data set of an optical microscope, Monash researchers have developed a machine-learning algorithm that can characterise graphene properties and quality within 14 minutes – much quicker than current methods. Presently the properties of graphene used in a product can only be determined after it has been manufactured.
Professor Majumder from Monash University’s Department of Mechanical and Aerospace Engineering, said the ‘game changing’ technology would allow hundreds of graphene or graphene oxide manufacturers globally to boost the quality and reliability of their graphene supply in quick time.
“Graphene possesses extraordinary capacity for electric and thermal conductivity. It is widely used in the production of membranes for water purification, energy storage and in smart technology, such as weight loading sensors on traffic bridges,” Professor Majumder said.
“At the same time, graphene is rather expensive when it comes to usage in bulk quantities. One gram of high quality graphene could cost as much as $1,000 AUD ($720 USD) a large percentage of it is due to the costly quality control process.”
“Therefore, manufacturers need to be assured that they’re sourcing the highest quality graphene on the market. Our technology can detect the properties of graphene in under 14 minutes for a single dataset of 1936 x 1216 resolution. This will save manufacturers vital time and money, and establish a competitive advantage in a growing marketplace.”
The research team, which also included Md. Joynul Abedin and Dr Mahdokht Shaibani (Monash, Department of Mechanical and Aerospace Engineering), and Titon Barua (Vimmaniac Ltd., Bangladesh), applied the algorithm to an assortment of 18 graphene samples – eight of which were acquired from commercial sources and the rest produced in a laboratory under controlled processing conditions.
Using a quantitative polarised optical microscope, they were able to identify a technique for detecting, classifying and quantifying exfoliated graphene in its natural form of a dispersion.
Mr Abedin said that in order to maximise the information generated from hundreds of images and large numbers of samples in a fast and efficient manner, the team developed an unsupervised machine-learning algorithm to identify data clusters of similar nature, and then used image analysis to quantify the proportions of each cluster.
He said the new method also has the potential to be used for the classification and quantification of other two-dimensional materials.
“The capability of our approach to classify stacking at sub-nanometer to micrometer scale and measure the size, thickness, and concentration of exfoliation in generic dispersions of graphene/graphene oxide is exciting and holds exceptional promise for the development of energy and thermally advanced products,” Mr Abedin concluded.
The study was published on Thursday in the international journal Advanced Science.